Table of Contents
Introduction to LLM Audio Sentiment Analysis
LLM audio sentiment analysis: Let’s consider a scenario where machines have a specialty; aside from grasping what we say, they can also recognize the feelings embedded in our tone. This is no longer a science fiction story; it is becoming a norm due to large language model audio sentiment analysis. With AI advancement, our skills to perceive human feelings through voice recordings in the present time are also enhancing. From bettering business interactions to changing the way mental health diagnosis occurs, this approach gives some hope for development cast scenarios. Let’s figure out how LLM audio sentiment analysis enhances the previous scenarios and why its use in emotion detection should not be underrated.
Importance of Real-Time Emotion Detection
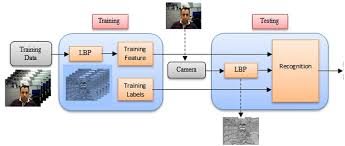
Timely interpretation of an emotion greatly benefits many industries, from customer service to mental health. Real-time assessments of emotions mean that companies can take action instantly. Hence, they can significantly add value to users and their experiences.
In medicine, understanding patients’ emotional condition can help decide on appropriate interventions at the right time. It also assists professionals in assessing the treatment’s outcome and revising the management plans, if applicable.
Children’s learning has excellent merits, too. Teachers can use children’s emotional states to deliver topics during lessons, making the classroom more exciting and compelling.
In addition, real-time emotion monitoring is proper during fights, arguments, or verbal negotiations because it reveals what each person feels throughout the discussion. This kind of realization increases and encourages understanding and compassion between parties.
Recognizing emotions in seconds opens up avenues for businesses with information that can help propel strategies in many industries.
The Technology Behind LLM Audio Sentiment Analysis
LLM audio sentiment analysis combines loudspeaker voice signature and speech recognition. This technology allows for computerized customer assistance while analyzing the client’s tone of voice.
Thanks to natural language processing (NLP), it would also integrate. LLM previously understood figurative languages in large-volume datasets. Hence, there is context and sentiment.
ML models also contribute very significantly. These learned models become more accurate with every new input of data they receive over time, which is very helpful in interpreting emotions accurately in real-time situations with several scenarios.
Another essential element for building these systems is feature selection. Instead of utilizing the entire audio of the speech signal, the system first locates the vowel’s or syllable’s center. Then, it uses other emotional sounds in the speech that can indicate the stress, joy, or sadness associated with it, which the system seeks out as a baseline feature.
All these technologies together create a robust system that has the power to build a voice emotion analysis system that can understand emotion through voice alone.
Applications of LLM Audio Sentiment Analysis
LLM audio sentiment analysis offers possibilities to extend the boundaries of multiple sectors. It can be employed in the customer service industry to determine the customer’s emotions during a call and instantly change tone and behavior.
Knowing how a patient feels can significantly improve the quality of care provided in medicine. A patient’s voice may reveal whether he is in pain or satisfied, thus helping the practitioner make necessary adjustments.
It also significantly concerns media and entertainment. Real-time audience engagement allows content creators to modify the story to evoke a strong response from the audience.
Educational institutions are also seeking alternative applications. Emotions are ingrained into a student’s personality; hence, capturing one’s feelings through classes can assist educators in altering their approach to increase a student’s interest and enhance learning.
Furthermore, social networks use such technology to evaluate how people react to fashion trends or specific events. Businesses instantly receive information on how the target audience feels about goods, enabling them to change the style of advertising promptly.
Challenges and Limitations of the Technology
While LLM audio sentiment analysis offers intriguing possibilities, it does have many barriers. One critical barrier is dealing with the multitude of different human emotions. Due to specific cultural and individual differences, emotions can be low, making their identification difficult.
Furthermore, background noise usually creates a problem in real-time analysis. Extraneous noise can disrupt emotion recognition algorithms in loud areas, even while speaking on the phone. This constraint can cause perception errors.
Another concern is the presence of significant variations in speech types and accents. Manner of speech can confuse the models trained on a specific dataset, so such approaches may not be practical in broader populations.
Furthermore, ethics also need to be considered. Unauthorized analysis of personal conversations raises privacy issues. Societal expectations of privacy remain a critical concern as technology advances in this area.
These challenges confirm that more research and development of LLM audio sentiment analysis are needed to improve its existing limitations by considering any further practical obstacles.
Future Developments and Potential Impact
There is a bright future for audio sentiment analysis using LLMs. As algorithms advance, we can expect better chances for emotion recognition. This breakthrough will allow businesses to customize their services more effectively.
Call centers come to mind where the customer service representative has emotional data information during a phone call. Their responses could vary based on the person’s mood on the other end of the line, which would provide enhanced satisfaction rates.
Mental health application features would also integrate such technology during therapy sessions, quickly transforming and enhancing them. Therapists can understand their patients’ underlying emotions more accurately through voice and speech pattern abstractions.
Other sectors that revolve around education may also have it easy. There would be voice analytics where teachers would learn how much students were engaged to make changes for better learning experiences.
Ethical issues will also be important as more research occurs in the field. The biggest concern would be how to protect data while still keeping up with technological advancements and how it can be used across various industries.
Conclusion
Audio sentiment analysis using LLM has grown significantly over the years. Technology is moving so fast that emotion recognition in real-time is also becoming valuable. Researchers and businesses, for instance, see the importance of sentiments in conversations, whether customer service calls or social media posts.
Businesses can now more clearly understand how a consumer behaves with tools that observe the customer’s feelings and tone of voice in subliminal ways. This function not only improves the user experience but also strengthens the bond with the customer.
However, considerable gaps remain, enabling us to attain “pure” accuracy and mitigate issues regarding data privacy. The research has targeted such problems, and ethical aspects have also been considered.
Looking into the future, the technique of audio sentiment analysis on an LLM basis also has profound implications for the field, whether it be communication with a patient or the development of advertising campaigns that emphasize emotional intensity. The future of humanity is quite bright with regard to these technological advancements.
FAQs:
What is LLM Audio Sentiment Analysis?
Answer: LLM Audio Sentiment Analysis is a technology that uses large language models to detect and analyze emotions embedded in speech, allowing machines to understand human feelings based on tone and voice.
What industries can benefit from LLM Audio Sentiment Analysis?
Answer: Industries like healthcare, education, customer service, and entertainment can significantly benefit from LLM Audio Sentiment Analysis by improving communication and service delivery.
How does LLM Audio Sentiment Analysis work in real time?
Answer: It uses voice recognition, natural language processing, and machine learning models to interpret emotions from speech instantly, providing timely insights into emotional states.
What challenges are associated with LLM Audio Sentiment Analysis?
Answer: Challenges include dealing with diverse emotions, background noise, speech variations, and ethical concerns about privacy during emotion detection.
How can LLM Audio Sentiment Analysis improve mental health diagnosis?
Answer: It enables therapists to understand underlying emotions in a patient’s voice, improving the assessment and treatment process in real-time.
What role does machine learning play in LLM Audio Sentiment Analysis?
Answer: Machine learning enhances the accuracy of sentiment analysis by allowing the system to learn and improve its predictions with each new input, adapting to various speech patterns.
How is LLM Audio Sentiment Analysis used in customer service?
Answer: It helps by analyzing the customer’s emotional tone during a call, allowing the service representative to adjust their approach to match the customer’s mood for better interaction.
What does the future hold for LLM Audio Sentiment Analysis?
Answer: The future includes more advanced emotion recognition capabilities, leading to better user experiences, more personalized services, and integration in various sectors like healthcare, education, and marketing.